How Big Tech Is Exploiting Content Creators, And (Trying To) Get Away With It
Diving into current courtroom dramas around AI, copyright, and the true meaning of “fair use.”
This post originally appeared on my Medium blog, katherineamunro.medium.com
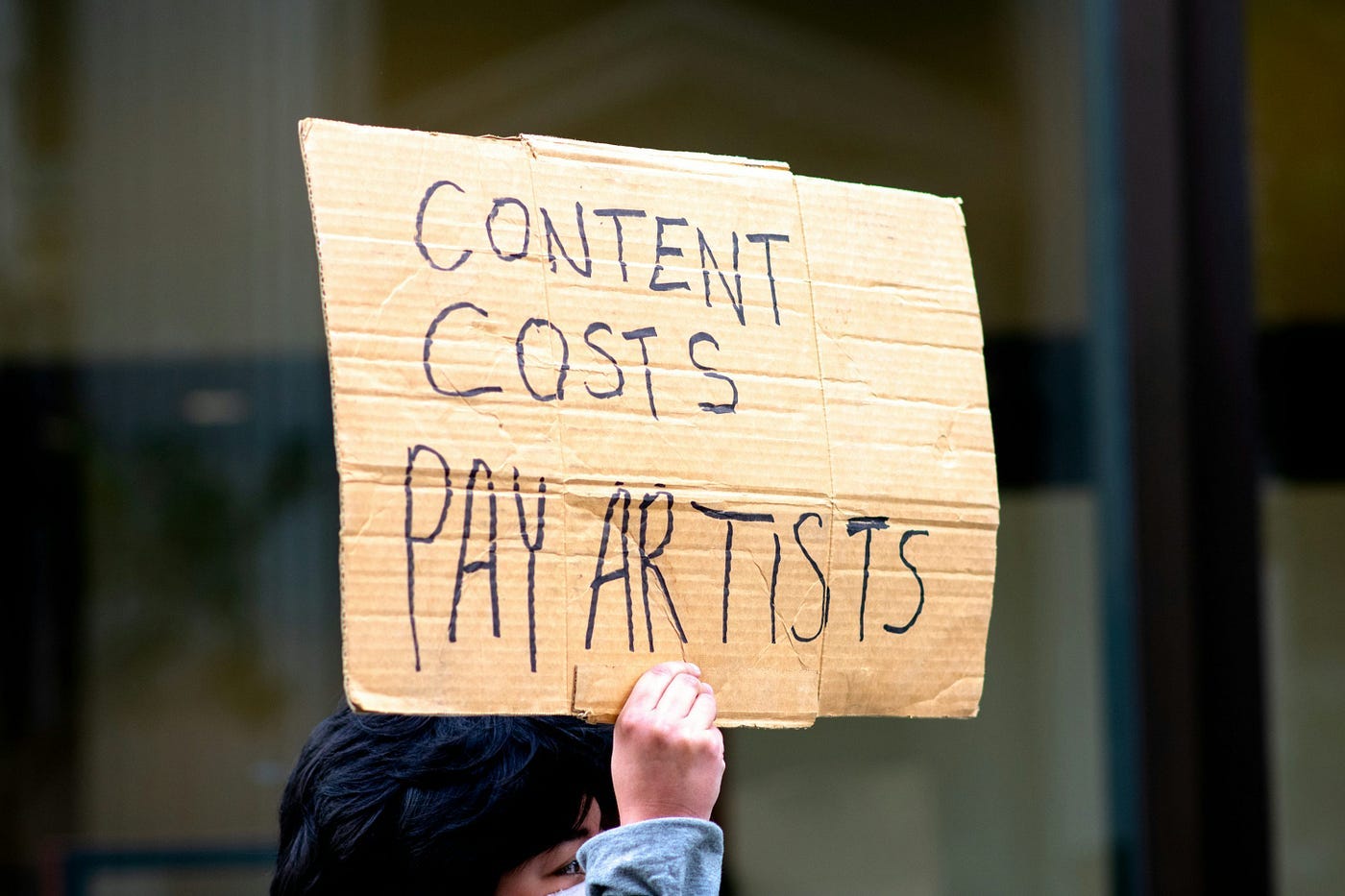
If you’re reading this, you’re part of the content creator ecosystem: either as a fellow writer, casual consumer or a Medium subscriber. You help keep the system running, which means you have a stake in the su…
Keep reading with a 7-day free trial
Subscribe to Beyond the Buzzwords, with Katherine Munro to keep reading this post and get 7 days of free access to the full post archives.